Advertisement
From the archive and in the news: How to cut through the 'noise' that hinders human judgment
Resume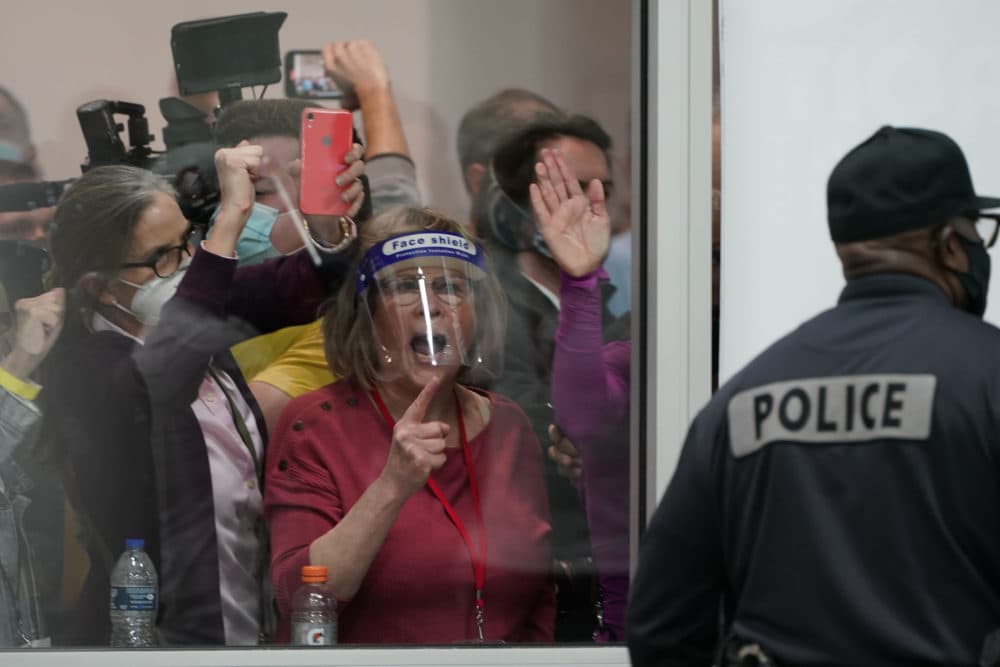
This program originally aired on June 01, 2021.
Nobel prize-winning economist and psychologist Daniel Kahneman has died at the age of 90.
In June 2021, we spoke with Kahneman, and his co-author Olivier Sibony, about their book, ‘Noise: A Flaw in Human Judgment.'
Guests
Daniel Kahneman, Nobel Prize-winning psychologist. Co-author of "Noise: A Flaw in Human Judgment." (@kahneman_daniel)
Olivier Sibony, professor of strategy and business policy at HEC Paris. Co-author of "Noise: A Flaw in Human Judgment." (@SibOliv)
Book Excerpt
Excerpt from "Noise: A Flaw in Human Judgment" by Daniel Kahneman, Olivier Sibony and Cass R. Sunstein. Copyright © 2021 by Daniel Kahneman, Olivier Sibony and Cass R. Sunstein. Reprinted with permission of Little, Brown & Company.
Transcript
Part I
MEGHNA CHAKRABARTI: Hi, everyone. It's Meghna here with a special "From the archives" podcast drop from On Point. Daniel Kahneman, one of the world's most celebrated economists, died this week at the age of 90. The Nobel Prize winner was one of the pioneers in a field that later became known as behavioral economics. His groundbreaking work showed that human intuitive reasoning is flawed.
In predictable ways, the predictability being the breakthrough. Kahneman was also author of "Thinking, Fast and Slow," a highly influential book that debunked a long-cherished belief in economics, that humans are rational actors. His work, along with others, showed both qualitatively and quantitatively that no matter how much economists want to believe it, human decision making is not a rationally driven process.
Kahneman was also a Holocaust survivor. His family was forced to wear the Yellow Star of David in occupied France before their escape. He later frequently said that his experience of the Holocaust was one of the things that drove his powerful interest in understanding the human mind. In June of 2021, we spoke with Kahneman and his co-author Olivier Sibony about their latest book.
It's called "Noise: A Flaw in Human Judgment." And it's about how, even though we're told to trust our judgment, that judgment is way more variable than we think it is. And it's also about how that variability, or noise, influences almost every part of our lives. So today, from the archives, we offer you our conversation with Daniel Kahneman.
I hope you enjoy.
CHAKRABARTI: This is On Point. I'm Meghna Chakrabarti. Back when I was 19 years old, I suddenly suffered from an autoimmune disease known as idiopathic thrombocytopenic purpura. It's a mysterious ailment where my immune system was attacking my own blood platelets, and it was pretty serious. The first doctor I saw said, "We don't really know what causes this, so I recommend waiting and watching, just don't do any major physical activity and it might resolve itself."
That seemed too passive. So the second doctor I went to, he said, "To reduce the autoimmune response, I recommend surgery to remove your spleen." That was very aggressive. So I asked, "What's the chance that procedure will work?" And he said, "50/50." Okay, so I went to a third doctor, and that doctor said, "You can take a complex series of steroids for several months and see what happens."
"Will it work?" I asked. And he said, "I don't know." Now I didn't mind the uncertainty because that is a fact in complex systems and the body is a profoundly complex system. What threw me was the wildly different solutions proposed by the three doctors, all for the same ailment in the same person.
And as a patient, I did not know how to cope with that variability. Now, I'm not a particular fan of n=1 examples or using one anecdote to describe an entire system. But it turns out that kind of variability is rampant. In the very professions, systems, and organizations whose judgment we are meant to trust the most.
It is a huge, costly, and often unnoticed problem. And it's a problem that Nobel Prize-winning psychologist Daniel Kahneman, Olivier Sibony, and Cass Sunstein write at length about in their new book, Noise: A Flaw in Human Judgment. And today, Daniel Kahneman joins us. Professor Kahneman, welcome to On Point.
DANIEL KAHNEMAN: Glad to be here.
CHAKRABARTI: And Professor Sibony, welcome to you as well.
OLIVIER SIBONY: Glad to be here as well.
CHAKRABARTI: Okay. So first, let me ask you, I did open up with that personal anecdote about the medical system. But Daniel Kahneman, how common or how much noise is in medicine, in decision making amongst doctors?
KAHNEMAN: The long and short of it is, there is a lot of noise.
Doctors don't agree with each other in many cases, and they don't even agree with themselves when shown the same set of tests on different occasions. So yeah. There's a lot of noise and there's a lot of noise in all professional judgment, not only in medicine, but wherever people make judgments you can expect to find noise and you can expect to find a surprising amount of noise.
CHAKRABARTI: A surprising amount. The thing about, I started with medicine because it's one of the systems that almost everyone has interactions with at some point, if not multiple points in their lives. Can you tell me, and Professor Sibony, I'll turn to you on this. Can you tell me a little bit more about what Daniel Kahneman was saying about how doctors even disagree with themselves when looking at the same set of information about a particular case?
How do we understand that? Yeah.
SIBONY: A typical example would be radiologists. And I suspect that radiologists are not better or worse than other doctors. It's just that it's easier to test radiologists because you can show them an X-ray that they've seen some weeks or some months ago and ask them, "What is this?"
And if they don't answer, of course, they cannot recognize the X-ray. Because they see a lot of X-rays. And if they tell you something different from what they had told you some weeks or some months ago when looking at the same X-ray, you know that is noise. Now, that is, by the way, a different type of noise from the one that you were dealing with in your example, Meghna, because this would be noise in the diagnosis.
Which is a matter of fact. You either have this bizarre disease that you were talking about, the name of which I could not remember, or you don't. At least your three doctors seemed to agree on the diagnosis. They disagreed on the treatment, which is already something you might find excuses for. Maybe there isn't an obvious treatment, maybe it's a very rare disease, we don't know.
But in the examples that we document in the book, they actually disagree on the reality of the diagnosis, of the disease that is present there. Which is a bigger issue, presumably.
CHAKRABARTI: Okay. Okay. So let's then step back here for a moment. I suppose we should actually begin with basic definitions here.
So Daniel Kahneman, when we're talking about noise in a system, we're not talking about individuals, but we're talking about the organizational level here. How do we define what noise is?
KAHNEMAN: We define noise as unwanted variability in judgments that should be identical, and that's the broad definition.
So your three physicians made judgments about the same case, and we would expect them to give identical answers. The fact that they're variable is an indication that something is wrong with the system.
CHAKRABARTI: And if I may, you're one of, not one of, I'd say you're probably the best-known psychologist in the world right now, okay, or at least one of them and your previous work Thinking, Fast and Slow, is an incredibly influential book here.
Does this interest in how human judgments across a systemic or organizational scale, it seems it must have, it naturally flows from your previous work, does it not?
KAHNEMAN: No, actually it didn't.
CHAKRABARTI: Ah, okay.
KAHNEMAN: My previous work, all my life, I've studied individuals, I've studied biases and not noise and I knew that noise exists, and everyone knows that when anything is a matter of judgment, people are not supposed to agree exactly.
So there is some noise. What turned out to be surprising was that some seven years ago, while on a consulting engagement with an insurance company, I discovered that there was much more disagreement than anybody expected, more than the executives expected, more than the underwriters whom we looked at expected, about by a factor of five, by the way, so it's not a small effect.
And that set me on this course, then Olivier joined me, then Cass joined us, and the book came out about seven years after.
CHAKRABARTI: And disagreement amongst, you were saying underwriters in particular in the insurance industry?
KAHNEMAN: Yes. So the way that we conducted the experiment, and we call that a noise audit.
Because it's quite general. You can conduct experiments like this in many cases. They constructed cases that were realistic but fictitious. You don't need to know the correct answer in order to measure noise. And then they presented the same cases to about 50 underwriters and each of them had to give a dollar value.
And the question that we asked ourselves and that we asked executives was, how much do they differ? And to give a sense of the magnitude of the difference, think that you pick two underwriters at random from those who looked at the same case. And by how much do they differ in percentages? That is, you take the average of their judgment, the difference, you divide the difference by the average.
How large is the difference? And I asked the executives that question. Not all executives, but a few. And since then, we, especially Olivier, have collected a lot of information on what people expect. People expect about 10% variation on quantitative judgment. That looks tolerable and reasonable.
You don't expect perfect agreement, 10% is tolerable. The answer for those underwriters was 55%. So that is not an order of magnitude, but that is qualitatively different from what anybody had expected. It raises questions about whether those underwriters were doing anything useful for the company.
CHAKRABARTI: I was just going to ask that, because if there's that much variability, what exactly are they doing, right?
KAHNEMAN: It is quite unclear, and I think there is a movement in insurance companies actually to take away that role of judging, of evaluating risk, to take it away from underwriters and to have them mainly as negotiators and to have the judgment automated or made centrally.
But at the time, that was a practice in that insurance company. Underwriters were actually setting dollar premiums. And the striking thing that really set this book in motion was not only that there was a huge amount of variability, but that the executives in the company had not been aware of it.
And that in fact, the organization did not know that it had the noise problem, right? So when you can have a problem of that magnitude, that people are not aware of, maybe there is something to be studied. That's what we did.
CHAKRABARTI: So then I think we need to understand more clearly and Professor Sibony, I'll turn to you for this.
How then does noise in your description of it differ from another word that Daniel Kahneman used just a moment ago from bias?
SIBONY: There is actually a very easy way to think about it. And it's to think of an example of measurement as opposed to judgment, it's easier to figure it. Suppose you step on your bathroom scale every morning, and, on average, your bathroom scale is kind.
It tells you that you're a pound lighter than you actually are. On average, every day. That's a bias. That's an error of a predictable direction. And on average, it's the error that your scale is making. Now, suppose that you step on your bathroom scale three times in quick succession, and you read a different number each time.
That is random variability of something that should be identical. It is noise. Now apply this to judgment, to see the difference between the bias, which is the average error. And the noise, which is the random variability in the judgments. Suppose that we're making a forecast of, say, what the GDP growth is going to be next year, or something like that.
If, on average, all of us who are making this forecast tend to be optimistic, that's a bias. We overestimate, that's an average error. But each of us is going to make a slightly different forecast, the variability between our forecasts is noise. So it's really quite simple, bias is a predictable error in a given direction, is the average error of a number of people or a number of observations by the same person.
Noise is a variability in those observations.
Part II
CHAKRABARTI: I'd like to focus with the two of you on one particular system that you write at length about and that is the judicial system. Professor Sibony, I wonder if you can help us understand what is the evidence that there is a great deal of noise or this unwanted variability, as you both called it, in judgments in the judicial system?
SIBONY: So there has been evidence for quite a while. One of the studies that we cite in the book goes back to the 1970s. And in that study, a great many judges, 208 judges, to be precise, looked at vignettes describing cases. So very simplified descriptions of cases, where you would expect pretty good agreement on how to sentence a particular defendant. Because the judges aren't distracted by the particulars of what happens in the courtroom or by the looks of the defendant or by any distracting information.
You would expect some consistency, perhaps not perfect consistency, but at least some consistency. And it turns out that on some of those cases, one judge would say 15 days and another one would say 15 years. On average, for a seven year prison term, that was the average given by the 200 judges, there was, if you were to pick two different judges, a difference of almost four years in what the sentence would be.
Which basically tells you that, if you're a defendant, the moment you walk into the courtroom, because you've been assigned to a particular judge, that has already added two years or subtracted two years from what would be otherwise a seven-year sentence. That is truly shocking. You would want, of course, the specifics of the case and the specifics of the defendant, and all the particular circumstances of a particular offense to be taken into account.
But the particular circumstances of the judge should not make a big difference to the sentence, and they do. And there have been quite a few other studies replicating and amplifying this finding, which basically tell you that who the judge happens to be, has a very large influence on the sentence.
Of course, we know that, but it's much larger than we suspect it is.
CHAKRABARTI: The legal profession has known this for quite some time, to your point, that they would, lawyers always talk about hoping to get assigned particular judges for their clients. But just to be clear, so these judges in the studies that you're talking about were given stripped down information about cases. So that ostensibly the factors that would normally contribute to bias from the individual judge were removed and yet we still saw this variability in sentencing. Is that what you're saying?
SIBONY: That is right. You can only expect that in reality the noise would be much worse than what we measure here, because these are stripped down cases. Where all the distracting information that could add and amplify the biases of the judge has been taken out.
CHAKRABARTI: So Daniel Kahneman, do we know why there was so much variability even in these controlled circumstances amongst these judges who, their profession is called judges.
We are told we are supposed to trust their judgment.
KAHNEMAN: Actually there is more than one source of noise. We distinguish three. So one source of noise are differences in the severity. And that's hanging judges and others, and that's the mean sentence, the mean of a lot of sentences that the judge gives differ across judges.
We call that level noise. Then there is the noise within a judge, that is elements that are like the weather, it turns out that sentences are more severe on hot days. It turns out that judges are more lenient when their football team just won a game. Those are small effects, but they are reliable effects.
It turns out that there is a lot of noise within a judge. Just as we were talking earlier about radiologists. And probably the largest source of noise is that the judges differ in how they see crimes. They have different tastes in crimes and different tastes in defendants. Some of them are more shocked by one kind of crime, others by another, and there are stable but idiosyncratic differences among judges, and that mysterious set of differences.
We call that the judgment personality, seems to account for much of the differences among judges in this judicial system and probably in other professional judgments, as well.
CHAKRABARTI: And is that judgment personality formed? It must be formed over time by ... judges. I don't know, that both their DNA and their personal experiences as they developed as humans.
KAHNEMAN: Absolutely. Except, we know very little about it. Because we know it's just like personalities. We don't expect personalities to be the same, but we actually expect to see the world in the same way. That is, I don't expect you to like the same things as I do. I don't expect you to behave the same way as I do, but I do expect you, when we're looking at the same situation, to see it as I do, because I see it correctly.
If I respect you, since I see the situation the way it is, I expect you to see exactly the same thing that I do. And that expectation is incorrect.
CHAKRABARTI: I am curious, though, about the second factor that you talked about, even if it's less influential, but the susceptibility of everyone, but in this case, judges sitting on the bench. To almost imperceptible things like the weather or whether their team won the game the previous night or not, because if the susceptibility to all manner of environmental inputs is part of the problem here, it seems as if it would be impossible to meaningfully reduce noise because it would require changing what makes us human, Professor Kahneman.
KAHNEMAN: It really depends on, to some, we must expect that noise will remain so long as there is judgment, because that actually defines judgment. A matter of judgment is a matter on which you expect some disagreement. So you're not going to resolve it completely.
But there are procedures, we think, that if followed by judges are going to make them less susceptible to variation. A source of variation I'd like to mention, by the way, is time of day. Judges and physicians are different in the morning and in the afternoon, when they're hungry and when they're not hungry.
So those are substantial variabilities.
CHAKRABARTI: Wow. So I want to talk more about some of those procedures in a moment, but Professor Sibony, let me turn back to you here for a second. Because I understand the intellectual utility of the kinds of studies that we're discussing here, regarding noise in the judicial system.
But at the same time, we're talking about judges looking at cases that have been stripped of a lot of detail, right? And isn't a part of what we are actually entrusting to judges is their discernment to come up with the right sentence, given the individual details of the cases that they are hearing. That, in fact, those details matter.
And then the judgments made by the people wearing the black robes should be trusted. Like, how much can we take these stripped-down studies and say that they are really pointing to something fundamentally flawed in the judicial system?
SIBONY: We have every reason to believe that if you add the real details that you see in a real courtroom, it would make the noise worse.
Now, there is an easy way to test that, which would be to actually take judges. I'm saying easy, it's actually not easy to do, but it's easy in principle. Which would be to take a number of judges and have them sit in separate boxes looking at the same trial. And at the end of that actual trial, having seen the real defendant and the real jurors and the real witnesses and so on, set a sentence.
We would see there what the real, that would be a real full scale noise audit, if you will, where you would see what the real noise is with real cases. To our knowledge, this hasn't been done. Because you can see it's a cumbersome experiment. But we are pretty convinced. I think there's good reason to believe that all the details you would see in an actual trial like this would only make the divergence between the judges worse than it is in the stripped-down cases.
CHAKRABARTI: Okay. So then help me understand Daniel Kahneman, have you, actually both of you, but Professor Kahneman, I'll turn to you for this. Have you spoken with judges about this and how do they respond when presented with this evidence of this sort of built in noise in their decision making?
KAHNEMAN: I have spoken with judges, but not enough to form an opinion, but a lot is known about the reaction of judges to discussions of noise and to guidelines that were introduced in an attempt to control the amount of noise by setting boundaries for different crimes.
And apparently, judges hated them. The guidelines were eliminated at some point, for reasons that are not pertinent to the case, but it turns out that judges are much happier about their job ever since, and clearly there is now more variability than there was. The situation in which there is a lot of noise is a situation that judges are entirely comfortable with.
They are comfortable with the situation; they don't know there is noise.
SIBONY: Yeah, and maybe if I may add something here based on my own anecdotal conversations with judges, here's how the conversation basically goes, right? You say there is noise, and you give them this evidence and basically, they shrug. They say yeah, that's the reality of making judgments.
Every case is different. So we're going to make different judgments every time. And then you ask them, okay, the same defendant is going to get a different sentence depending on whether he's assigned to you or to the judge next door, and they say, "Yeah, that's life." And then you ask them, "What if the same defendant got a different sentence because his skin is of a different color?"
And they say, "No, that would be completely unacceptable." And then they realized that we have a very different level of outrage when we can explain the cause of a discrepancy, when we can identify a bias and when it is noise that we cannot identify. There isn't any obvious reason why we should feel it's completely acceptable for these differences to appear for reasons we do not understand, whereas it is totally unacceptable. And I think we would all agree on that, for them to appear because of reasons that we do understand.
And that's what we're trying to point out when we raise the question of noise in the judicial system. Why do we tolerate large differences that are caused by noise when we would not tolerate them if they were caused by bias.
CHAKRABARTI: Okay. You mentioned, both of you mentioned guidelines. And Professor Kahneman, can you just elaborate a little bit more about within the criminal justice context, what you meant by guidelines?
KAHNEMAN: There was a commission set up, I think in the late 1970s or 1980s to discuss, to assign to each crime as defined in the law, to assign a range of sentences and judges were strongly discouraged from going outside those, outside that range. They were allowed to do it. So there was discretion.
But clearly the guidelines had a great deal of effect and the variability of sentences for any given crime indeed diminished. So those were, that's just part of the definition of a crime in this range of sentences that are allowed to go with it. That's a guideline.
CHAKRABARTI: Okay. So the reason why I want to ask you about that is because you do talk about the importance of creating, I would say, the right kind of guidelines to reduce noise in organizations and systems.
Because the one that pops up in my mind right now, which I think has been deemed to be something of a failure, is exactly what you're talking about. Mandatory minimum sentencing, for example, in drug crimes, that you're right. The judge's discretion was removed from them, with mandatory minimum sentences.
And the part of the logic behind those mandatory minimums was to reduce variability in sentencing. However, what we saw, one of the outcomes of that was also many people being sentenced to extremely long periods of incarceration for relatively minor drug crimes. So there is still some sort of systemic judgment that emerged with those guidelines of the mandatory minimums, which actually made the problem of achieving justice even worse.
Professor Sibony, how do you find what the right guidelines are without introducing a whole another set of problems? Because in trying to reduce unwanted variability and reaching more identical solutions, you can get a bunch of identical solutions that aren't the right ones.
SIBONY: Absolutely. And that would be bias, right? So that would be an average error. If you think that the proper sentence for a given crime is one year in prison and you set a mandatory minimum that is ten years, you have reduced noise. Because everybody will get 10 years, but you have created a lot of bias, because everyone has a sentence that is 10 times worse than it should be.
And so that elevates the question of what the proper sentence should be to a debate, that has to take place in the U.S. Congress, as opposed to being a decision that is being made separately by hundreds of judges every day. Now, it's interesting that when it becomes a problem of bias, when it becomes a problem of the overall decision being made at the wrong level, it is at least a debate we can have.
And we can say, three strikes and you're out, is terrible. Mandatory minimum sentences are terrible. We can have that conversation. When that decision is being made quasi randomly by judges all around the country every day, the noise is very hard to control, and it leads to many bad decisions as well.
Not all the decisions are uniformly bad, but the randomness is in itself very bad. That's the difference between bias and noise. One is much easier to see. It's much easier to counteract. It's much easier to discuss and to combat. The other is all over the place, and if you don't do a noise audit to measure how much noise there is, you can't even see it.
Part III
CHAKRABARTI: we're trying to reduce, figure out how to reduce noise. And Professor Kahneman, before the break, we were talking about guidelines and mandatory minimums in the judicial system as one perhaps flawed way in trying to deal with the noise problem in sentencing. And I just wanted to quickly hear your thoughts about that.
KAHNEMAN: We should not say that guidelines are a bad idea because some guidelines were poorly designed. In this case, clearly there was a great deal of bias in the setting of the guidelines. For example, they distinguished among different kinds of drugs in a way that penalized crack cocaine, relative to other drugs.
Those are poorly designed guidelines, bias guidelines, which will perpetuate bias rather than eliminate error. But you can design good guidelines. And the point about guidelines, and here I echo something that Olivier was saying earlier. The point about guidelines is that you can see them. You can discuss them.
They're out there. Noise is something that you cannot see, and you cannot respond to appropriately.
CHAKRABARTI: What other types of guidelines, just sticking with the judicial system for one more minute here, what other types of guidelines that you suggest in the book might be applicable here? If the guidelines are defined as guidelines on sentencing, that's the kind, and that's the type, the only type, we would say we have ideas about procedures, about ways of thinking about the crime.
And a defendant and a particular case that we think might reduce noise, but in terms of guidelines, sentencing guidelines, designed sentencing guidelines is what there is, what is available, I think.
CHAKRABARTI: Okay, so then tell me about what you just, tell me more about what you just said about the other solutions for the judicial system.
KAHNEMAN: The general concept that we propose is a concept that we call decision hygiene, and the term is almost deliberately off putting. It's to remind you of what happens when you wash your hands. And when you wash your hands, you kill germs. You don't know which germs you're killing, and if you're successful, you will never know.
And it's a sort of homely procedure but it's extremely effective. And we have been scouring the literature and what we know to construct a list of decision hygiene procedures. And one of them, just to give you an example, the most obvious one is to ask several individuals to make judgments independently, because that will reduce noise mechanically.
When you take several forecasters, and you average their forecast, the average forecast is less noisy than the individual forecast. And we know exactly by what mathematical amount, we have cut down on the noise. So that is another procedure. And there are several others that Olivier, I'm sure, can talk about it, at least as well as I can.
SIBONY: Meghna, just to come back to guidelines for a second, there is one field in which guidelines have made a great difference, and that's medicine. You were talking as we started this conversation about the disease for which clearly there weren't guidelines, or if there were, your three physicians were not aware of them, sadly for you.
But in many fields, guidelines have made a big difference. One example that many people will have encountered is that when a baby is born, to determine if the baby is healthy or needs to be sent to neonatal care, you use something called the Apgar score, where you apply five criteria, abbreviated A, P, G, A, and R, and you give this little baby that is one minute or five minutes old, a score between zero and two on each of those five criteria. And if the total is six or less, the baby has a problem. If the total is seven or more, the baby is healthy. And that has reduced massively the noise and therefore the errors in the treatment of newborn babies. It's a great example of a guideline that actually works.
It's a fairly simple guideline, but it's not something, one dimensional, like a minimum sentencing guideline for a particular crime. It takes into account multiple factors, but it makes sure that different people will take the same factors into account, and we'll take them into account in the same way, so it reduces noise.
Those kinds of guidelines, when they're well thought through, can actually make a big difference.
CHAKRABARTI: Okay. So you also mentioned a noise audit briefly in the last segment there. Professor Sibony, how would you define what a noise audit is?
SIBONY: So a noise audit is not a way to reduce noise. It's what you need to do first.
It's a way to measure noise. So when we gave the example of the underwriters or the example of the justice system, these are noise audits where you get a feel for how large noise is. And the reason you need to do that is that as Danny was pointing out, we don't imagine that people see the world differently from how we see it, and therefore we can't imagine that there is as much noise as there is. Because if I'm a judge, I never hear what another judge would have sentenced this particular defendant to, because each defendant is unique.
And if I'm a doctor and I look at an X-ray, I never imagined that another doctor looking at the same X-ray would see something different from what I see. So a noise audit makes this visible and tells you exactly how much noise there is in your system.
CHAKRABARTI: So there are, those are just, that's a small taste of the quite extensive writing that you have in the book about ways to reduce, to know about, assess and reduce noise in various systems and organizations, but I'd like to just push to one potential solution and get both your opinions on it. And that is if you want to reduce variability entirely. Unwanted variability. You take the human condition out of it. I'm of course, I'm talking about technology.
People are actively trying to create AI systems that achieve exactly what you're talking about. Take various inputs and come up with the same solution every single time. Professor Kahneman, is that a desirable way to reduce noise?
KAHNEMAN: In some, there have been many studies that compared rules and algorithm to human judgment, and in many of these studies human judgment comes short. And one of the main reasons, and that we know that humans come short, is because of noise. Because humans are noisy and algorithms are not, you present the same problem to an algorithm twice and you get the same answer, which is not the case when you do it with humans.
We can expect that algorithms, when the information is codable. So there are some conditions for algorithm to work well. You need codable information, you need a lot of data, and you need a choice about the criteria that you're applying so as to eliminate bias to the extent possible.
And then you can have a system that is likely to do better than humans. And in the judicial system, there is an example. And the example is the granting of bail, where a recent study using AI techniques, I forget the number of millions of cases that they looked at, but it's a very large number, they were able to establish that an algorithm would actually perform better than the judicial system, in the sense that it would both reduce crime and reduce unnecessary and unjustified incarceration.
So at least in that domain, there is clear evidence that an algorithm can do better than people.
CHAKRABARTI: If the algorithm has been eliminated of the potential biases in its creation, right? Because sticking with the judicial system, I was reading several years ago about how, for example, in Washington, D.C., and this is also being used everywhere, you were talking about bail, and this is actually regarding the AI use in parole.
That prosecutors were using an AI assessment system to decide whether or not to put parole on the table for a particular defendant. And defense lawyers had discovered that AI system was making risk assessments based on factors that included whether or not a person lived in government subsidized housing or whether they had ever expressed negative attitudes about the police. And it seemed that there was ample opportunity for bias to actually be built into that AI system, which was a problem.
KAHNEMAN: Absolutely.
SIBONY Absolutely. No question. No question about that.
And that's something to be absolutely worried about. But just to be clear, one biased algorithm, or two, or ten. Do not mean that all algorithms must be biased, and algorithms have a big advantage over humans, which is that, again, we can have that conversation. We can measure whether an algorithm is biased.
We can have ProPublica audit the algorithm and tell you that the algorithm has this particular bias or does not have that particular bias, and then it can get fixed. Of course, that must happen. It doesn't happen by magic. It takes action from people who worry about it and who make sure that algorithms improve.
But at least we can have that conversation. A biased judge, a biased human being, is very difficult to spot, because of the noise in the judgment, in part because of the noise in the judgments of that person. No judge is so consistently biased that you would be able to indict that particular judge for being biased.
CHAKRABARTI: Yeah. Looks like a little internet instability there, but Daniel Kahneman there's something I've been wanting to ask you all hour here, because we're talking about how noise can be proliferated and amplified through a system. But of course, that system is made up of individual human beings.
And I wanted to hear from you about how this actually does connect to your previous, pathbreaking research about individual judgment. Are there things that individuals can do regarding their thinking, to reduce their contribution to the noise?
KAHNEMAN: There are, our ideas on this matter is really quite straightforward, and it applies even to individuals' decisions not only to systems.
It applies to singular decisions, to strategic decisions that people make, and our argument is quite straightforward. If decision hygiene procedures work in repeated cases, there is every reason to believe that they will apply, as well, to unique or singular cases. So decision hygiene recommendations are applicable to any judgment that people make. On the argument, which actually, Olivier, the one who had that phrase that we're very grateful for, that the singular event is a repeated event that happens only once. So that everything that we say about noise as repeated events is actually applicable to individual judgments.
CHAKRABARTI: But more specifically, from your book, Thinking, Fast and Slow, where you describe the different types of thinking, don't you, aren't there certain types of thinking that achieve exactly what you're saying, that there are actually people who maybe intuitively are noise reducers?
KAHNEMAN: We doubt that. Because, and the reason that we doubt that and the connection with Thinking, Fast and Slow, is that intuition is very rapid.
Intuition doesn't make efficient use of all the information. So our major recommendation in that context, and it's an important one, is that intuition should not be eliminated from judgment, but it should be delayed. That is, you want not to have a global intuition about a case until you have made separate judgments, until you have all the information.
Whereas the human tendency is to jump to conclusions. Jumping to conclusions induces noise.
CHAKRABARTI: We only have a few minutes left. It saddens my heart because I have so many more questions for both of you, but there's one more system I want, I wish to explore with you just briefly. And that is governance or political systems.
And particularly, let's just look at the United States. Because I feel like we are in a moment where noise is the point. We have very influential people in our political system who have said, I'm thinking of Steve Bannon, for example, who said that his goal was to flood the zone with BS, essentially.
How, is there anything in your book that we as citizens can apply to reducing the noise and improving the decision making in a political system, in the American political system?
KAHNEMAN: I don't think there is anything specific that is to be applied. If people thought better, in general, and make better judgments and better decisions, we might be better off, but the differences in the political system are closer to issues of bias than to issues of noise, and bias and convictions based on very little evidence and on poor evidence, those are political problems. And to those, we have no solution to offer that I know of. Perhaps Olivier can think of something, but I have not.
SIBONY: Unfortunately, no. Unfortunately, not. There is one thing though, which is not a solution, but which is part of the problem that we discuss at some length in the book. Which is that groups and any forum, including social media, in which people are going to interact in a group, tend to amplify the random noise that comes from the opinion of a few people at the beginning of a process.
So any system, and I'm thinking mostly of social media, in which people are going to be part of an echo chamber, is going to add to the randomness in the positions that people have eventually and is going to add to the polarization of those positions.
CHAKRABARTI: If Olivier Sibony and Daniel Kahneman especially don't necessarily have a solution for the chaos inducing noise in our political system, I don't know who would.